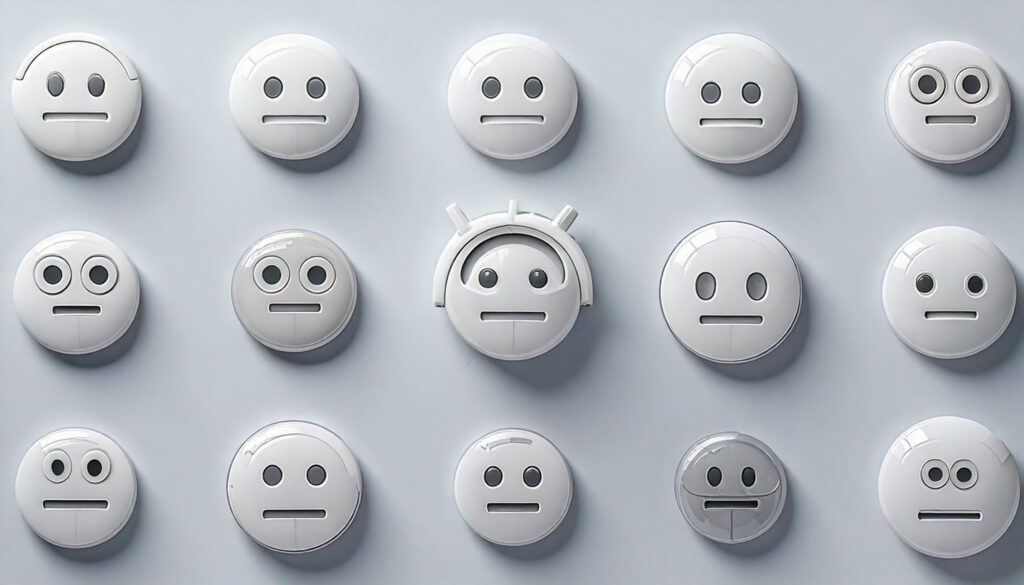
Part 3 (of 3) in the Designing UX Surveys That Work series.
Part 1 highlighted the “Must Do’s” for impactful survey design, while Part 2 tackled the art of crafting unbiased questions to ensure accuracy in your data. Now, in Part 3, we’re taking a closer look at the wider world of bias traps—those sneaky pitfalls that can distort your survey results—and sharing strategies to help you identify and minimise them for more reliable insights.
Even if you’ve crafted neutral questions, bias can still creep into your research in other ways. From sampling bias to confirmation bias, these factors can distort your results and lead to inaccurate conclusions. Let’s examine these traps and how to avoid them.
Sampling Bias: when your participants don’t represent your users
Sampling bias occurs when the participants in your survey don’t accurately represent the larger user group you’re trying to reach. For example, if you’re designing for a national or global audience but your survey primarily attracts respondents from one region, your results may not reflect the diversity of user needs, limiting your study’s validity.
How to mitigate it:
- Define your sample scope: As discussed in Part 1 (), use online calculators to determine statistical relevance (e.g., the percentage of the market or total users you need to survey).
- Recruit diverse participants: Use multiple channels such as email lists, social media, and user panels to engage a broader and more representative audience.
- Monitor demographics during recruitment: Track factors like age, location, and gender to ensure diversity.
- Be transparent in your reporting: At the end of your insights report or presentation, outline the scope and limitations of this round of surveys. Highlight what has been achieved, what gaps remain, and how you plan to address those gaps in future studies.
Non-Response Bias: when certain Users don’t participate
Non-response bias arises when certain types of users are less likely to participate in your survey, skewing the results. For instance, busy professionals might ignore lengthy surveys, leaving responses from users who may not represent your core audience.
How to fix it:
- Keep surveys concise: Respect participants’ time by limiting the number of questions to the essentials.
- Offer incentives: Small rewards, such as gift cards or discounts, can motivate users to participate, particularly those who are harder to reach.
Cultural Bias: when it doesn’t translate across demographics
Cultural bias occurs when a survey assumes a specific cultural context or uses language that doesn’t resonate with all participants. This can alienate certain user groups or lead to misinterpretation of questions.
How to avoid it:
- Localise content: Adapt your survey language and examples to suit different cultural groups.
- Test with diverse participants: Pilot your survey with your colleagues or users from various cultural backgrounds to identify and address cultural blind spots.
- Avoid slang and colloquialisms: Stick to clear, universally understood language and steer clear of culturally specific references.
Confirmation Bias: when you see what you WANT to see
Confirmation bias occurs when researchers interpret data to support their pre-existing assumptions or hypotheses. For example, a designer involved in creating a product prototype may subconsciously craft survey questions to validate their design decisions.
How to avoid it:
- Document assumptions: Clearly outline your assumptions and hypotheses in your research brief.
- Seek contradictory evidence: Actively look for data that challenges your preconceived notions.
- Pilot test your survey: Have someone outside the core design team review your survey for objectivity, as discussed in Part 2 ().
Bias Detection Checklist: spotting and fixing issues before launch
Here’s a quick checklist to identify and resolve bias in your surveys:
- Are the questions free of hidden assumptions via leading or loaded questions?
- Avoid questions that assume a precondition and maintain a neutral position to minimise any bias.
- Is the language neutral?
- Avoid emotionally charged words (e.g., “amazing,” “terrible”) or leading phrases (e.g., “Don’t you think…”).
- Are all answer options balanced and inclusive?
- Ensure that scales or multiple-choice options cover the full spectrum of possible answers, including negative feedback.
- Is the survey answered by enough percentage of people to represent the target group for statistical relevance?
- During the planning process, define the percentage of/target number of participants required to respond. Ensure this is sufficient to represent your target audience accurately.
- Have you randomised your answer options?
- Randomising answer options prevents primacy or recency bias, ensuring fair representation of all choices.
- Have you done a pilot test of your questions?
- Having someone else proof-read the questions ensures baseline objectivity before launch.
Turning data into insights
Once you’ve accounted for these common bias traps, your survey data will be much more reliable. The final step is to analyse the results with an open mind and translate findings into meaningful insights to share. Use the data to inform user personas, prioritise features, or identify pain points—but always cross-validate with other research methods like User interviews or usability testing.
Surveys are a powerful tool in any UX researcher’s arsenal, but their effectiveness hinges on thoughtful design and execution. By applying these best practices, you’ll gather insights that truly reflect your users’ experiences and drive better design decisions.
Designing UX Surveys That Work series:
- Part 1: Crafting Effective UX Surveys: The Must Do’s
- Part 2: The Don’ts in Survey Design: Eliminating Bias for Better User Insights
- Part 3: Avoiding Common Bias Traps in UX Surveys
Recommended further reading
- Just Enough Research by Erika Hall
- Surveys That Work: A Practical Guide for Designing and Running Better Surveys by Caroline Jarrett
- Survey Design Best Practices by Nielsen Norman Group