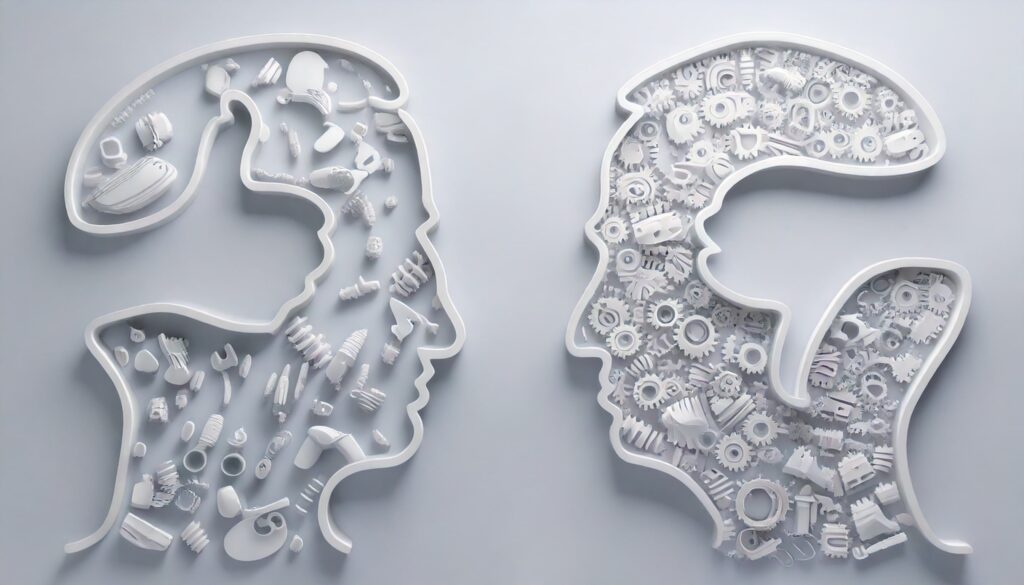
Part 2 (of 3) of the Designing UX Surveys that Work series
Unintentional bias
Surveys are one of the go-to methods in gathering quantitative data in UXR (UX Research). They help us measure trends, validate user personas, or gather insights on sentiments for improvements at scale. In Part 1, we covered the essentials (the Must Do’s) of defining a survey’s purpose and setting a strong foundation for success. But there’s a catch: without actively being conscious of common traps, we unintentionally introduce bias. And one of the most common culprits? Our questions. To design effective surveys, we need to avoid writing questions that lead participants to specific answers or influence them unintentionally. In this post, we’ll explore how to craft unbiased survey questions and design the most professional surveys.
Why bias in surveys are big concerns in UX
Imagine this: you’re conducting a survey to understand how users feel about your product’s new feature. You ask, “How much do you like the new feature?” Most users respond positively, and you conclude that the feature is a success. But here’s the problem—the question itself assumes the user likes it. This is a classic example of a leading question. As an analogy, in courtroom dramas on tv, you’ve seen a lawyer object to a cross-examination of her client with a shout “Leading the witness! Your honour…”. The same exact principles applies here.
Bias in surveys doesn’t just skew your data, it can lead to poor design decisions. Worse, it can erode trust with your Users. If your questions feel manipulative, participants may disengage or provide inaccurate answers. It’s key for UX designers to take designing surveys with best practices as we would for designing product experiences. After all, taking surveys is itself an experience , isn’t it?
What are “Leading” and “Loaded” questions?
Leading questions – based on assumptions
A leading question subtly (or not so subtly) nudges respondents toward a specific answer.
- These questions often make assumptions or include emotionally charged language
- Typically leading questions channel and direct the respondent into an answer with our own bias
- They can invalidate your entire study
- Leading questions can appear in many different types.
Example of a leading question:
“When you use X feature, how useful do you think it is?”
The above might sound innocent enough, but it assumes that the participant has actually used the feature (they might not have)
- It also influences them to “agree” on some level that it’s useful when they might not feel that way
- It’s leading the User to make conclusions that don’t reflect reality.
How to fix it:
Reframe the question neutrally. For example:
“How would you rate the ease of use of feature X? If you haven’t used it yet, skip to the next question.”
By balancing out the positive the the negative, the question is neutral and direct. Also providing a 5-point scale rating (2 levels of negative, 1 neutral, 2 level of positive) this is easily going to yield higher fidelity of data. The added bonus is that this can used to generate better charts/graphs during the insights report generation phase.
Interconnected statements – leading questions
This type of leading question uses unnecessary context to influence the participant to answer more favourably when they may not have wanted to. For example:
“Our most active customers use X feature every time they log in. How many times do you use this feature?”
How to fix it
This example could cause the participant to feel guilty or uncomfortable about their experiences with your product. Remove the context and stick with the straightforward question.
Scale-based leading questions
When you write a question that uses a scale (or equivalent), the options to choose from need to be balanced. Otherwise, you risk skewing the results towards one side of the scale. For example:
- Extremely satisfied
- Very satisfied
- Somewhat satisfied
- Somewhat dissatisfied
- Very dissatisfied
How to fix it
This example shows there are more positive options than negative ones – leading to an increased probability that you’ll gather more positive responses.
Add a neutral response in the middle and an even number of positive and negative options on either side.
Loaded Questions
Loaded questions embed assumptions or presuppositions that force participants into a particular frame of mind, often without them realising it. Typical characteristics are:
- It assumes a fact about the participants in the question itself – presupposes a truth that has not been confirmed
- It compels the participants to accept a certain premise as true
- sort of like a trick question – no matter how they respond, they’re forced into a response they might not agree with
- Opinions and responses can suffer as a result.
Example of a loaded question:
“Have you stopped using X feature?”
- If the participant said “no”, they’re stating they still use the feature;
- If they say “yes”, they’re stating they used to use the feature
- if the participant never used the feature in the first place, they can’t appropriately answer the question.
How to fix it:
Remove the assumption and ask it instead. For example:
“Have you ever used this feature before?”
Another example of a loaded question:
“Why do you think this vendor is the best, given their higher prices and mixed reviews?”
- The question assumes there are issues with the vendor, pushing the person to explain their preference
- Bias of the participant is more likely to be revealed.
How to fix it:
Remove the assumption and stay neutral on the topic. In this scenario an open question would work great. For example:
“In your own words, could you best describe how you feel about this vendor, including its prices for its services?”
Tips for writing unbiased survey questions
1. Keep it neutral
Use neutral language that doesn’t push respondents in any particular direction. Avoid words with strong positive or negative connotations, like “amazing,” “terrible,” or “obviously.”
2. Ask 1 question at a time
Double-barrelled questions can confuse participants and lead to unreliable data.
Example of a double-barrelled question:
“How satisfied are you with our app’s features and customer support?”
Split this into two separate questions:
“How satisfied are you with our app’s features?”
“How satisfied are you with our customer support?”
3. Provide balanced answer options
For multiple-choice questions, ensure that the answer options cover the full spectrum of possibilities. Avoid framing options that lead respondents toward a particular choice.
Example of unbalanced options:
“How would you rate our app?”
- Excellent
- Good
- Fair
This leaves no room for truly negative feedback. Instead, use a balanced scale:
- Very Poor
- Excellent
- Good
- Neutral
- Poor
4. Pilot test your survey
Before rolling out your survey, test it with a small group. Here your co-workers (not necessarily from UX only) can work well. Ask them if any questions felt confusing, biased, or difficult to answer. Their feedback can help you refine your survey.
The impact of bias-free surveys
When your survey questions are fair and neutral, you’re rewarded with data that truly reflects your participants’ experiences and opinions. Much of the bias seeping into our questions are unintentional and typically human. The best practices we’ve covered here – it is a craft we UXers must learn to get conditioned to and constantly improve to do better.
Eliminating bias doesn’t stop there. Even with neutral questions, other forms of bias—like sampling bias, response bias, and cultural bias—can still creep into your research and distort your data. In part 3 of this series (coming up) , we’ll explore these broader bias traps and provide strategies to identify and mitigate them.
Designing UX Surveys That Work series:
- Part 1: Crafting Effective UX Surveys: The Must Do’s
- Part 2: The Don’ts in Survey Design: Eliminating Bias for Better User Insights
- Part 3: Avoiding Common Bias Traps in UX Surveys
Recommended further reading
- Just Enough Research by Erika Hall
- Surveys That Work: A Practical Guide for Designing and Running Better Surveys by Caroline Jarrett
- Survey Design Best Practices by Nielsen Norman Group